In her senior thesis, Yael Stochel '22 studied why different species of butterflies developed similar wing patterns. Photo by Damiano Pappadà.
By Rina Diane Caballar
Artificial intelligence may not seem like an obvious tool for studying butterflies. But for Yael Stochel ‘22, machine learning provided exactly what she needed to understand animal behavior in the wild.
Stochel studied two species of butterflies that evolved millions of years apart but have nearly identical wing patterns. Both species of butterfly are toxic to birds, and scientists have shown that despite being evolutionarily distinct they developed similar wing patterns as a strategy to mutually deter predators.
But the patterns are so similar that they create a puzzle: how do these butterflies mimic each other closely enough to deter predators, but not so closely that they confuse each other and interfere with mating? Stochel’s senior thesis research showed that while birds have sharper vision than butterflies, they are unable to distinguish between the two species. Butterflies, on the other hand, are usually able to tell each other apart.
Stochel’s unique approach was to apply the tools of machine learning and computer vision to understand how birds and butterflies — not humans — see wing patterns. “The goal,” according to Stochel, “was to apply computer science to that system of mimicry and use machine learning and computer vision to model the system that exists in the wild.”
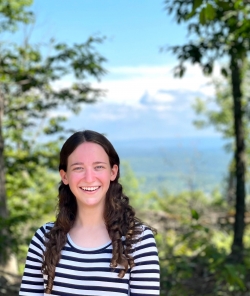
Stochel used the ButterflyNet neural network, which uses deep learning to analyze thousands of images of butterflies and has proven, with 86 percent accuracy, that the wing patterns of these butterflies mimic each other. Building upon ButterflyNet, Stochel used machine learning classification algorithms to train additional neural networks to approximate how birds and butterflies learn to identify wing patterns.
Imageomics Institute at the Ohio State University, which answers biological questions through interdisciplinary methods, also contributed to the project. The butterfly experts at Imageomics helped Stochel eliminate the misleading images of hybrid butterflies from her search and concentrate on the relevant species.
“Yael’s thesis is a fascinating blend of computer science and evolutionary biology,” said Stochel’s advisor, Class of 1877 professor of zoology Daniel Rubenstein. “Her algorithms, training and testing methods, and color analysis showed that machine learning can make the invisible visible to science, thus helping scientists gain insights into challenging evolutionary problems.”
Stochel also received guidance from computer science professor Olga Russakovsky and support from Christopher Lawrence, a Ph.D. student in Princeton’s ecology and evolutionary biology department.
According to Lawrence, Stochel’s determination and openness to feedback were two qualities that helped her create an outstanding research project. “Yael was always seeking to try things and figure out what was possible or needed before coming to me and Dan to ask questions.” She was always “open to feedback, learning and sharing,” he said.
Stochel’s senior thesis not only demonstrates the importance of computer science tools in exploring biological questions, but also helps researchers to better understand how butterflies might be affected by rapidly changing habitats. One result of the work, Stochel said, is to understand how “the changing environments in the regions where these butterflies live potentially pose a threat to the stability of the mimicry system.”
Stochel graduated with high honors, earning her degree in computer science with a certificate in environmental studies. She was elected to membership in Sigma Xi, the scientific research honor society, and awarded a prize for outstanding computer science independent work.