PNI/CS Special Seminar
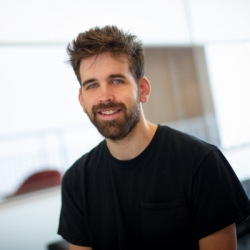
Human cognition is characterized by a remarkable ability to transcend the specifics of limited experience to entertain highly general, abstract ideas. Efforts to explain this capacity have long fueled debates between proponents of symbol systems and statistical approaches. In this talk, I will present an approach that suggests a novel reconciliation to this long-standing debate, by exploiting an inductive bias that I term the relational bottleneck. This approach imbues neural networks with key properties of traditional symbol systems, thereby enabling the data-efficient acquisition of cognitive abstractions, without the need for pre-specified symbolic representations. I will also discuss studies of perceptual decision confidence that illustrate the need to ground cognitive theories in the statistics of real-world data, and present evidence for the presence of emergent reasoning capabilities in large-scale deep neural networks (albeit requiring far more training data than is developmentally plausible). Finally, I will discuss the relationship of the relational bottleneck to other inductive biases, such as object-centric visual processing, and consider the potential mechanisms through which this approach may be implemented in the human brain.
Bio: Taylor Webb received his PhD in Cognitive Psychology and Neuroscience from Princeton University, where he studied with Michael Graziano and Jonathan Cohen. He is now a postdoctoral research fellow in the Psychology Department at UCLA, working with Hongjing Lu, Keith Holyoak, and Hakwan Lau. His research is focused on the question of how the brain extracts structured, abstract representations from noisy, high-dimensional perceptual inputs, and uses these representations to achieve intelligent behavior. To better understand these processes, his work exploits a bidirectional interaction between cognitive science and artificial intelligence. This involves both the use of techniques from AI to build models of higher-order cognitive processes (e.g., metacognition and analogical reasoning) that are grounded in realistic perceptual inputs (e.g., images and natural language), and the development of novel AI systems that take inspiration from cognitive science and neuroscience to achieve more human-like learning and reasoning.
To request accommodations for a disability please contact Yi Liu, irene.yi.liu@princeton.edu, at least one week prior to the event.